Human beings visually sense the world around them, using their eyes and brain. Computer Vision is a branch of Machine Learning and Artificial Intelligence aimed at providing the same ability, or even better than humans, to computers or machines.
Computer vision uses a set of techniques such as automatic extraction, contextual analysis, etc., to understand and determine useful information from an image or a sequence of images, i.e., a video. Algorithms are developed based on mathematical theories to build systems capable of automated visual understanding.
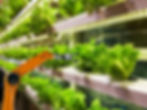
Detection of Plant Diseases
Automated plant disease detection is hugely beneficial since it reduces the tedious job of monitoring a large crop area. The most significant advantage is that it can detect the symptoms very early as soon as they appear on the leaves. This allows farmers to take steps early on and prevent other crops from getting damaged. Genetic algorithms are used to segment the images, which is a pivotal point in detecting leaf diseases.
Use of Deep Learning to Grade Crops
The use of deep learning techniques has taken automation to the next level. They are here to remove the human aspect altogether. The algorithms resort to automatic learning. The model is presented with a set of perfect crops and another set of defective crops. Self-adjustment allows the algorithm to classify any new crop submitted to the system successfully. Whenever the new presented crop looks like one of the sets, it is automatically classified as such. This method has proven to be extremely fast, reliable, and consistent. It is a state-of-the-art solution that is highly recommended for any classification example of this kind.
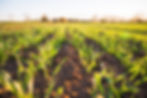
Precision Agriculture and Computer Vision
There is immense scope to utilize computer vision and artificial intelligence to reduce the costs for farmers. Some examples in this regard are the detection of fruit diseases from cellphone camera images allowing timely action, reduction in the use of herbicides by applying them only where there is a need, detection of water losses and leaks by using high-end infrared cameras, etc. Cameras can be installed above the conveyor belts to classify crops in real-time; greenhouses can be tracked to look for plant growth, and animals can be tracked using cameras to monitor their behavior giving a timely alert and a call for action.
Conclusion
Computer Vision algorithms are expected to become more complex over time, taking into account the current outdoor fields and weather conditions for which they are required. There are no off-the-shelf solutions and algorithms available at the moment to cater to the tremendous amount of variance encountered in weather, soil, and other environmental conditions that are incredibly erratic. Artificial Intelligence and Machine Learning Engineers are working on developing robust algorithms that would work in all situations and meet the current demand and solve the vast amount of problems the farmers have to face in the fields.