The use of Computer Vision in automated vehicles can prove to be a game-changer. We can design and develop advanced next-generation vehicles equipped with the ability to overcome hindrances and obstacles, ensuring the people's safety on board. Such cars can transport people to their destination without any human aid or intervention.
The idea of such vehicles is quite fascinating, but it is still in the development stages. It will require some time to mature before seeing such cars on urban roads filled with traffic. This is because the stake is relatively high. Even a small imperfection can prove to be catastrophic, leading to fatal accidents and loss of life.
Computer Scientists, researchers, and developers use computer vision algorithms and technology in autonomous vehicles to ensure the safety of the passengers and the pedestrians, along with the surrounding traffic. Let us discuss a few use cases of computer vision in automated vehicles:
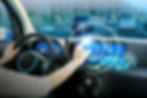
Three-Dimensional Map Creation
Creating 3D maps will allow self-driving vehicles to capture visual information dynamically. Such vehicles are equipped with cameras that capture live footage. Computer Vision algorithms applied to this footage can create 3D Maps. With these maps, the vehicle can determine the surroundings with precise obstacle detection and opting for the best possible route for fast access.
3D Maps also allow accident predictions and instant deployment of airbags. This safety measure makes such vehicles safe and reliable for road deployment.
Object Detection and Classification
Computer Vision technology makes self-driving cars classify and detect objects with high accuracy. Vehicles can use LiDar sensors, which use pulsed laser beams to measure distance. This data coupled with 3D maps allows better detection of traffic lights, cars, etc. This allows the vehicle to make decisions on the fly analogous to an actual human driver.
Training of Algorithms
The computer vision technology can gather a lot of data using the cameras and sensors such as the traffic conditions, road safety, maintenance, blockage, etc. This detailed information can help the vehicle to make vital decisions with speed.
They will also be used to train the deep learning models further. An example is this case is that if the Deep Learning model is shown a thousand images of traffic signals, it can automatically detect one when it sees it while driving. It also improves object classification that is vital for the correct operation of the car.
Computer Vision Aided Low Light Mode
During the night time, self-driving vehicles use different algorithms for processing the images and videos than the daylight. Low light photos can be blurry, and the data might not be sufficient enough for accurate driving.
As soon as the computer vision technology detects the low light conditions, it shifts to the low light mode. The reliance is turned more on LiDar sensors, thermal cameras, and the High Dynamic Range sensors. They allow for the creation of high-quality images and videos.
The night time performance has long been a big challenge for such vehicles. Computer Vision algorithms and technology ensure that the vehicle is not just made for day time use but remains functional around the clock.
Conclusion
Computer Vision can make self-driving vehicles much more intelligent, reliable, and self-reliant. If we can correctly implement the technology and algorithms, the possibilities are endless. We can land on uncharted territory if we truly harness the potential of this incredible technology.